2015 | Mapping crop diseases
Globally, crop diseases result in significant losses in crop yields. To properly target interventions to control crop diseases, it is important to map diseases at a high resolution. However, many surveys of crop diseases pose challenges to mapping because available observations are only proxies of the actual disease, observations often are not normally distributed and because typically convenience sampling is applied, leading to spatially clustered observations and large areas without observations. This paper addresses these challenges by applying a geostatistical methodology for disease incidence mapping.The methodology is illustrated for the case of bacterial wilt of banana (BWB) in the East African highlands. In a survey using convenience sampling, 1350 banana producing farmers were asked to estimate the percentage yield loss due to bacterial wilt. To deal with the non-normal distribution of the data, the percentages were classified into two binary variables, indicating whether or not the disease occurred and whether or not the yield loss was severe. To improve the spatial prediction of disease incidence in areas with low sampling density, the target variables werecorrelated in a logistic regression to a range of environmental variables, for which maps were available. Subsequently, the residuals of the regression analysis were interpolated using simple kriging. Finally, the interpolated residuals were added to the regression predictions. This so-called indicator regression kriging approach resulted in continuous maps of disease incidence. Cross-validation showed that the method yields unbiased predictions and correctly assesses the prediction accuracy. The geostatistical mapping is also more accurate than conventional mapping, which uses the mean of observations within districts as the predicted value for all locations within the district. The maps were also spatially aggregated to district level to support regional decision-making. The analysis showed that the disease is widespread on banana farms throughout the study areaand can locally reach severe levels.
Download article Mapping crop diseases using survey data
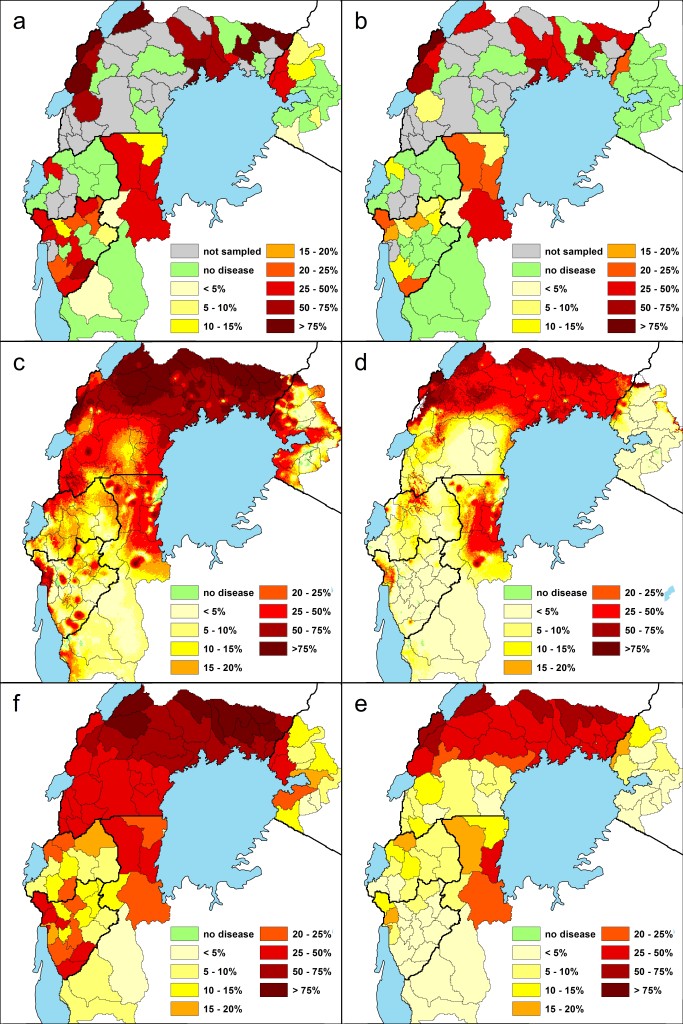